AI is everywhere! How did we get here and what’s in it for me?
-
Posted by Quest Editor
- Last updated 12/12/23
- Share
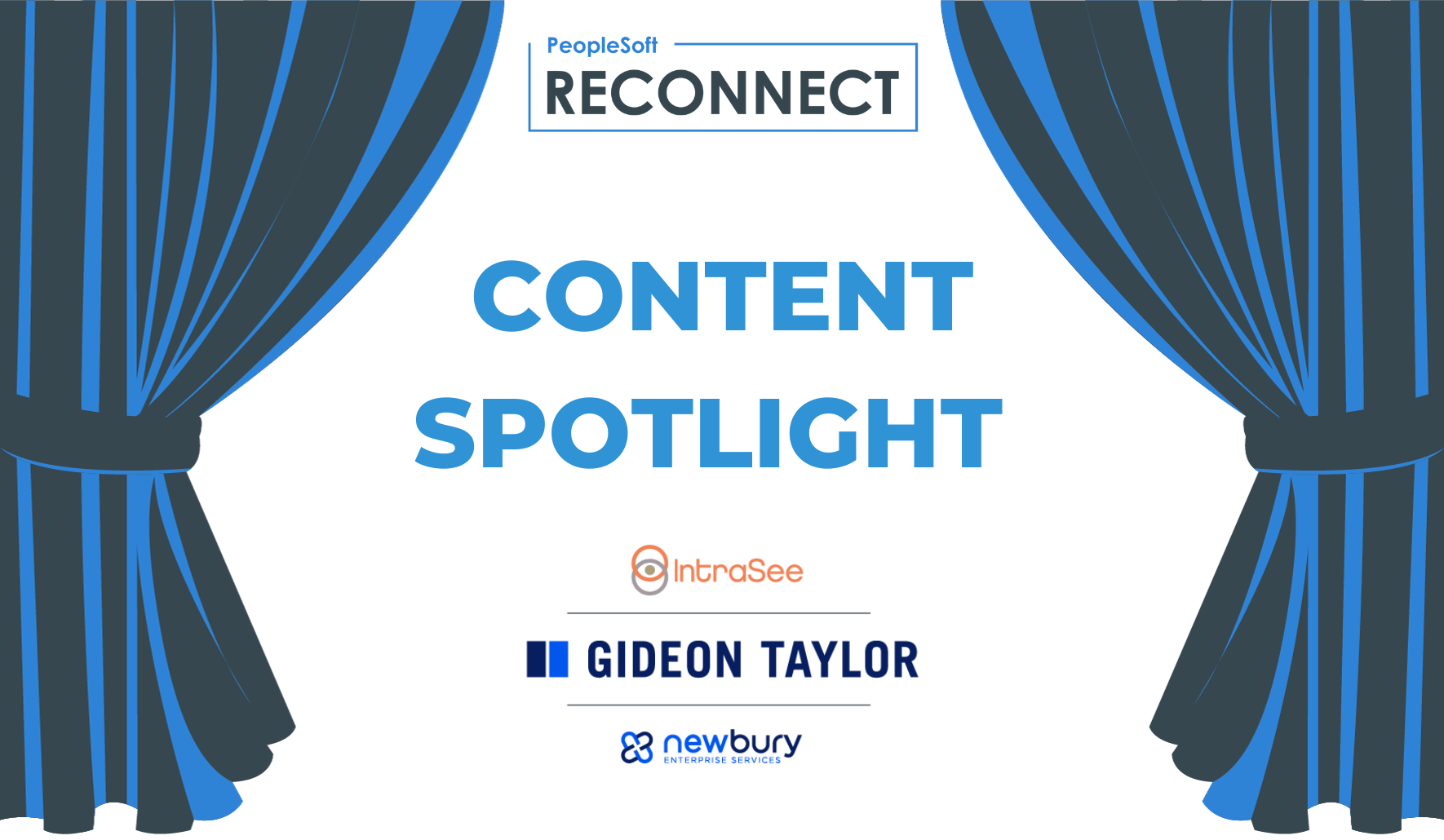
AI is everywhere! How did we get here and what’s in it for me?
AI has captured the attention of the world. It’s the subject of social media posts, blogs, and news stories. Why is that? What got us to this point? And what does this mean for the future? Most specifically, how can you leverage the capabilities of AI for your enterprise?
Andrew Bediz, Managing Director of the IntraSee division at Gideon Taylor, answered these questions in a presentation at the RECONNECT Deep Dive virtual event. Andrew has been in the industry for 25+ years, including a stead at PeopleSoft in the late 90s.
Gideon Taylor as a company has been in the Oracle ecosystem for more than two decades. Their goal is to meet the needs of their customers today and to help them take the next right step for tomorrow. With emphasis on their conversational AI product, Ida, for PeopleSoft, Gideon Taylor’s well-rounded knowledge and experience with AI makes them an ideal presenting sponsor for our deeper conversation regarding AI and your enterprise.
What is AI?
According to a recent survey, 83% of companies claim that AI is a top priority in their business plans.
How did AI get to this point of fever pitch? Primarily, two major advancements paved the way: Deep Learning and Large Language Models with Generative AI. Essentially, Deep Learning helps with understanding, and LLMs and Generative AI help to scale the technology.
The objective of AI is the ability to be more human-like in its decision making. The human brain reasons by grouping like concepts together. Then it uses inference to process information it’s never seen before. For example, as a human, you could spend your entire adolescence in North America. You learn how to drive on the right side of the road and know what stop signs look like. Then, as an adult, you take a trip to the UK. Even though you’ve never experienced driving on the left side of the road and following European road signs, you can do it because of your ability to infer.
AI researchers developed a way to mimic the human brain using a neural network of nodes. Giving this neural network specific inputs with their matching outputs allows it to start to understand. It’s the same as a student being given homework assignments by a teacher. That homework contains questions (the input) and the student learns how to answer (the output). When that student takes a test at the end of the semester, the questions are in a form they have never seen before, but the student reasons and uses inference to deliver the right answer. A diagram of this process is below:
In AI, we teach the model that there are several ways to ask a question (input). This is called “training the model”. We constantly feed new information into the model over time, and the model learns—just like a student building on their own knowledge through books.
The information that feeds an AI model can come from several different sources. It may receive data from labelers, software, user feedback, or data.
Labelers are basically the teaching assistants. They look at the raw data going into the model and help the AI to understand the information. They tutor it to help with comprehension. These are the humans helping along in the AI journey.
For your purposes, you may have data in your enterprise system—from ticketing, interactions, mobile app, etc.—or from software that can input nodes into your conversational AI.
This process, recreated with technology through models, is called Deep Learning.
Large Language Models, on the other hand, are largely to blame for the frenzy that is AI in today’s world. LLMs multiply the number of nodes of input into the billions.
GenAI stands for Generative Artificial Intelligence. To generate means to create. When AI is generative, it is creating its own answers. In the case of something text-based, like Chat GPT, the AI is using word probabilities to make up answers. It really isn’t creating something truly new, but rather reshuffling the old. There are some major pitfalls with this technology as it applies to the enterprise. Generative AI has the potential for hallucination, in which the system is convinced it’s correct—based on bias or prior learning that was inaccurate—even as it returns something untrue.
The best use cases for enterprise-wide generative AI are:
Co-piloting — GenAI suggests the user review the suggestion and approve it instead of AI doing the work automatically.
Content — In areas of marketing, training, or sales, GenAI can wordsmith, revise, edit, or create imagery for you but you’ll still have final say.
Generative AI for text is illustrated below:
Everything with AI is math. It’s a series of probabilities. For the example above, based on probabilities, “problem” is the most likely next word after “Houston we have a…”.
With any AI, you have to pay close attention to how it’s trained and who trains it. Are those sources credible? GenAI simply reshuffles the words that someone else wrote, so are those words free from bias?
It’s important to note that the initial purpose of Chat GPT was not to be helpful. It was to be convincing. The point was to emulate a person so well that you can’t tell that it’s not a human. After its impressive showing of human imitation, Chat GPT was so powerful and intriguing that the second wave of intention was to make it correct.
It’s good to keep in mind that Chat GPT starts with a goal to convince you of something and then comes back with guard rails to try to make it right.
For a snapshot of Large Language Models, we’ll look at GPT4 below:
GPT4 is based on 45 GB of training data, primarily sourced from public places such as Wikipedia, Reddit, Twitter, etc. The 175 billion parameters (10x more than GPT3.5) are the input nodes discussed earlier. GPT4 has gone through a heavy dose of reinforcement learning, in which human beings have reviewed outputs to reinforce whether they were correct or incorrect. This is one of the reasons ChatGPT was free—so that you and other people could reinforce the models. It takes around 100 days and $100 million each time the model is trained or retrained.
This is a large challenge for the enterprise. The idea that your institution could run a Large Language Model is almost impossible. You probably cannot allocate $100 million to a training run. Instead, you’re going to use someone else’s.
The companies that can afford LLMs are big tech players such as Oracle, Microsoft, and Google. Your opportunity is to leverage those companies’ LLMs for your enterprise.
Why should you use AI in your enterprise?
In terms of marketing statistics, the graphic below shows public opinions which undoubtedly will set expectations at work or at school:
How can you take those marketing stats and leverage them for your organization? Clearly, customers do not want to touch base with a helpdesk. They’d prefer messaging.
Where can you use AI in your enterprise?
The graphic below shows the areas in which many organizations are leveraging AI for their enterprise.
One of the common areas of use is prediction and analytics. As an example, imagine it’s time for benefits enrollment and your employees are grumbling about how to pick the right plan. By using persona data such as marital status, number of dependents, location, or age cohort, AI can suggest that other people have found maximum happiness with a suggested selection.
Computer vision gives the ability to get meaning out of images and videos. It can detect objects. You may see this in security software to trigger alerts.
Infrastructure scalability is growing in popularity. It relates to right-sizing your infrastructure based on seasonality. AI can do this automatically based on trends in the data.
Unstructured meaning detects meaning from data found in loose text. It can be advantageous for combing through resumes and matching to best fit jobs.
Anomaly detection can identify patterns that are out of the norm, and send alerts to help protect you from security threats.
Virtual assistants interpret the user’s language into intent. The use cases are countless. It helps the user via Helpdesks, conversational UI, analytics, reports, processing transactions, and more.
Large Language Models for Your Enterprise
While LLMs are advantageous, we’ve already discussed that they can deliver false information. If your user searches for an answer via conversational AI in your enterprise, they will receive an answer—but it may not be your company’s correct answer.
For example, an employee asks the conversational AI how much per diem they’ll receive for an upcoming trip. If you haven’t supplied an answer to the AI, it will still return an answer based on it’s general understanding. The results will be a popular answer from other sources. It’s probably not correct.
The solution to this problem is to input your company’s policies, or house rules, into the AI.
In traditional machine learning, you would input the information through examples of identification. The downside is all of the human effort that goes into teaching the machine.
However, if generative AI can generate the correct output, there’s less time to be invested by humans. That is a bit of a trap because the common way to use LLMs is a technique called RAG (Retrieve, Augment, Generate).
Through automatic prompt engineering, the user queries an LLM and then inputs specific data and knowledge. The LLM takes that input and generates the output.
A problem surfaces because of the specific data and knowledge that the AI requires to generate an accurate answer. Whatever time was saved on the front end is lost during the data and knowledge submission. Plus, that data must be high-quality, timely, and unchanged in order for it to work.
The idea of using GenAI to reduce the human burden of these systems is simply not so clear cut. It’s actually even more important to put the energy into the knowledge being fed to the generative AI. No matter what you do, AI isn’t going to help you and use your data unless you play a role in the process. This is important to note because some vendors will build the AI for you, but it won’t be based on your data, information, titles, and acronyms. The results will be much more generic. AI is garbage in, garbage out. You’ll get from it what you put into it.
At Gideon Taylor, the professionals create a dedicated model for each client. The client trains the model by using a specific tool available from Gideon Taylor.
Case Study of Gideon Taylor Customer: Seneca
The Challenge
Seneca deployed a chatbot for prospective students, but the chatbot only helped with admissions. It was ill fit for current students due to its lack of personalization and integration to enterprise systems such as PeopleSoft. Given Seneca’s large student body and significant international numbers, serving this volume at all hours was a major challenge.
The Solution
Seneca deployed Sam and started ramping up users around 4,000 per month starting in August 2020. Sam could handle prospective student questions and authenticate within the enterprise systems. Today, Sam can answer almost 700 questions personalized to the user’s role, including data and transactions from PeopleSoft and Salesforce.
Sam’s accuracy rate is 96%. For comparison, the typical human resolution rate on the first call is only 74%. In a single month this year, Sam provided more than 50,000 answers with 44% of those occurring outside core business hours. This generated almost $1 million in ROI opportunity for that month.
Related to Sam’s success, Seneca decided to get rid of their Microsoft bot and turned off their Salesforce bot. They found it more advantageous to have one bot that did everything, instead of individual knowledge-based and website-crawling bots.
Case Study Takeaway
Breadth is key. Sam was able to answer questions from ten different categories, which ebbed and flowed over time. If they had chosen to focus on just financial aid or only career knowledge, they would have failed to answer thousands of submitted questions—missing out on millions in ROI opportunities.
From Andrew’s perspective, Chat GPT has been popular because of its breadth. Users love that it can answer anything they ask. In positioning your enterprise for success, it’s imperative to build breadth in. If not, you’ll struggle with adoption and receive too little feedback to achieve success.
You can also watch how Gideon Taylor’s leverages their bot, Ida, in this video from 50:40 to 56:45, or watch the full RECONNECT Dive Deep 2023 presentation for more details.